‘Evolutionary’ AI Tricks Classic Video Game Q*bert Into Delivering Points Overdrive
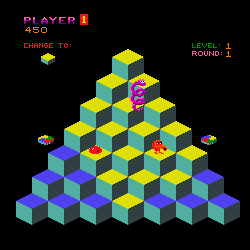
New research demonstrates evolutionary techniques may hold promise for training artificial intelligences of the future
An artificial intelligence system has discovered unusual ways of racking up large numbers of points in the classic 1980s Gottlieb video game Q*bert, after being used in an experiment by German researchers.
Researchers at the University of Freiburg found their artificial intelligence discovered two “particularly interesting” solutions to an Atari console version of the popular arcade game after being trained with sets of random parameters.
One of the solutions involves repeatedly killing an adversary by jumping off the edge of the game’s pyramid, while the other exploits a previously unknown bug.
The research, published in a new paper by Patryk Chrabaszcz, Ilya Loshchilov, Frank Hutter, analysed the performance of a type of AI that uses evolutionary tactics to discover improvements.

Evolutionary tactics
Such Evolutionary Strategies (ES) AIs are less well known than those trained using reinforcement learning (RL), the most famous of which include Google’s DeepMind and IBM’s Watson.
Chrabaszcz, Loshchilov and Hutter concluded evolutionary techniques perform better than RL in some cases, and that the two methods could be combined to good effect.
Evolutionary AIs develop by feeding random parameters into large number of policy networks and then adding incremental changes – also randomised – and measuring which resulting networks deliver better results.
Suicide ploy
In one case, the researchers found that instead of trying to finish the game’s initial level, a network instead found it could kill one of its enemies over and over to gain large numbers of points.
“The agent learns that it can jump off the platform when the enemy is pght next to it, because the enemy will follow,” the researchers wrote. “Although the agent loses a life, killing the enemy yields enough points to gain an extra life again. The agent repeats this cycle of suicide and killing the opponent over and over again.”
Bug delivers points
In the second result highlighted in the paper, the AI uncovered a previously unknown bug in the version of the code used for the trials.
After completing the first level, the agent begins to jump from platform to platform in what seems like a random manner.
“For a reason unknown to us, the game does not advance to the second round,” the researchers wrote. “The platforms start to blink and the agent quickly gains a huge amount of points (close to 1 million for our episode time limit).”
They added that the bug doesn’t always appear, and in fact in 22 out of 30 trials the AI yielded a low score.

AIs of the future
One of the game’s original developers confirmed that the AI seemed to have hit on something that wasn’t intended to be in the game.
“This certainly doesn’t look right, but I don’t think you’d see the same behaviour in the arcade version,” Warren Davis, who worked on the arcade version of Q*bert, told the BBC.
The paper includes links to videos of the first and second strategies being put into effect.
The researchers argue the results show both evolutionary and reinforcement learning networks have different stregths and weaknesses, meaning improved techniques could be arrived at by finding ways of bringing the two together.
“We… expect rich opportunities for combining the strengths of both,” they wrote.
Put your knowledge of artificial intelligence (AI) to the test. Try our quiz!